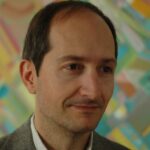
Machine-learning Optimized Design of Experiments
Interview with Tommaso Dorigo about the MODE collaboration
As reply to the JENAA call for Expression of Interest Tommaso Dorigo and his colleagues proposed a program for Machine-learning Optimized Design of Experiments – MODE. Their main target is the use of differentiable programming in design optimization of detectors for our research field. The first Kick-off Meeting took place last September and they just published a preprint of a short article on their research plan on INSPIRE which will be published by Nuclear Physics News International. In this interview, Tommaso Dorigo will tell us more about MODE and next steps.
Can you explain more about the program and aim of MODE?
For over a century now, physicists have designed instruments to detect elementary particles, and radiation in general, exploiting cutting-edge technologies, and in some cases developing entirely new ones. As the complexity of the apparatuses and of the required tasks grew, so did our inventiveness. This has brought a stream of new developments, which culminated in the past two decades with the construction and operation of giant detectors like ATLAS and CMS, which are mind boggling instruments.
Precisely because of their complexity the design of such apparatuses has followed well-defined paradigms, which have served us well until now, and guided us toward robust design choices and well-established techniques. However, those choices are not – and cannot be – perfectly aligned with our true experimental goals. The reason is that the task of optimizing the design of these apparatuses is absolutely super-human, as it requires the study of configuration spaces of hundreds, if not thousands of dimensions. In fact, a global optimization is usually not even attempted: we use as success-metrics simplified surrogates of our real goals, and this potentially results in huge losses in performance.
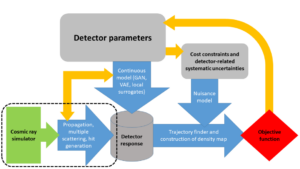
A proposed pipeline for the optimization of a muon tomography apparatus (Figure taken from the article on the MODE collaboration published in Nuclear Physics News International, March 2021).
Yet today we can, in principle, rely on artificial intelligence for the exploration of those hugely complex parameter spaces. Differentiable programming techniques allow us to navigate through them, if we provide the right interfaces and construct models of the whole experiment, from the simulation of the events of interest, particle interaction with matter, detector response and reconstruction, and inference extraction. This is very hard, I am not hiding that. But we need to start doing it. MODE has the goal of proving how such a path can be undertaken, to realign our experimental choices with our true goals, and to vastly improve the effectiveness of future detectors.
But MODE is not specifically targeting those giant multipurpose detectors for fundamental physics – quite the contrary, in fact. MODE researchers are starting this ambitious program by working towards smaller-scale practical applications of particle detectors, such as proton therapy or imaging with cosmic muons. In these areas, the detectors are relatively small and their geometry is way less complex than those of particle colliders. Nevertheless, design optimization is far from trivial also in these applications. In fact, the first practical implementation of the MODE program may well be in one of these areas, where the typical timescales from design to operation are relatively short.
At the JENAS 2019 the call for EoI was issued and you came up with the MODE program. Did you and your colleagues already work on this topic before, or did you just start after this event?
I have worked on machine-learning-driven optimization of physics measurements in the past, but my idea of applying the techniques developed in that context to the design of instruments was born while sitting in board meetings of accelerator physics coordination. There, I observed that the design of new detectors for future colliders was being proposed and starting, by the hands of colleagues with decades of experience in instrumentation, without any consideration for the elephant in the room, AI. In 20 years, the extraction of information from detector signals will be entirely automated and in the hands of much more complex and performant algorithms than those in use today. This means that constructing devices with the same paradigms as before is doomed to be enormously suboptimal.
Of course, and fortunately, I am not the only one who realizes this, and in fact efforts in the use of advanced computer science techniques to the optimization of detectors and instruments have started to appear in the past few years. Some of the MODE members are in fact leaders in this area of research, with some important publications already produced. With the help of these colleagues, we thus formed the MODE collaboration, to provide the ground where to build the required interfaces for a more systematic approach to detector design.
To what extent does your collaboration represent the three communities Particle, Astroparticle and Nuclear Physics ?
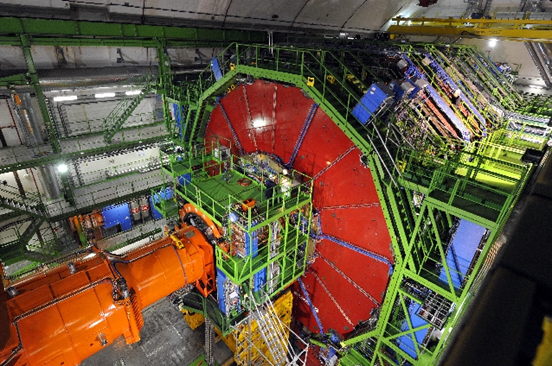
A view of the CMS experiment at CERN. The complexity of modern particle physics experiments is too high to allow for human-driven optimization. Or, better put, the space of design choices is so vast that the potential for improvement in relevant metrics (discovery potential, data quality) is huge. (Credits:CERN)
Our group for now is small, but highly motivated. I cannot cite everybody here, but MODE includes physicists who are experts in machine learning and already working for calorimetry optimization (Jan Kieseler, at CERN, Fedor Ratnikov, at HSE University and Yandex Data school, and colleagues at National Research University Moscow), track reconstruction (Mia Tosi, at University of Padova), inference extraction (Pietro Vischia, at UCLouvain, and Giles Strong, at INFN-Padova), and muon tomography (Andrea Giammanco, at UCLouvain) – all those tasks are important use cases for MODE, and are not specific of HEP. And we have computer scientists with experience in collaboration with physicists (Atilim Gunes Baydin, at Oxford University, and Gilles Louppe, at Université de Liege); plus Ph.D. students in HEP (Hevjin Yarar and Lukas Layer, at INFN-Padova). But MODE tries to be as inclusive as possible, because of the extremely challenging nature of its research program. We need the interest of everybody who wants to extract information from devices that work by detecting radiation in any form, and therefore it is only natural to look beyond the playground of some of us, which is HEP. Hence we have started to involve colleagues from the astroparticle physics and nuclear physics community, as well as neutrino physics, by inviting them to take part to the advisory committee of a workshop we are organizing, which we hope will be the first of a series, and by asking them to chair sessions there and take part. In conjunction, we are advertising our research plan within those communities, as we believe that our studies will benefit them just as much as HEP.
It is important to realize that particle detectors can be improved quite significantly in their performances by studying even very simple choices, such as moving detection elements around. Last year I did an exercise with a simply designed detector, MUonE, which will be built to reduce a theoretical uncertainty on the g-2 muon anomaly. The experiment aims to measure the differential muon-electron elastic scattering with layers of silicon impinged on by a beam of muons at CERN, and is very simple – so simple that I could study it with a fast simulation and demonstrate that with some optimization a factor of two gain in the relevant metric could be achieved without increase in cost or complexity. A publication ensued, and the collaboration is now using my results for an improved design. But this is just an example.
How can ECFA, NuPECC and in particular APPEC support your activities?
Help in making the MODE research program more visible and known within the communities is certainly important – we have indeed already benefited from the offer of publishing a short manifesto in the Nuclear Physics News International journal. Also, we presently have no explicit funding for MODE, so support for the organization of a yearly workshop will be very welcome.
You plan a MODE Workshop on Differentiable Programming this autumn. What are the aims of the workshop and who should participate?
The workshop aims at making these techniques more widely known, as well as at creating a stable bridge and a communication ground with the computer science community. Anybody who realizes that these tools, which today power artificial intelligent devices all around us, are needed for fundamental physics research in the future should consider coming, listening, or giving a contribution. I mention artificial intelligence in everyday life objects (cellphones, self-driving vehicles, targeted ads, spam filters, etcetera) because these things have changed the paradigms in our society, but this was only possible because it was economically favourable to invest in creating the right interfaces for the problems to be solved. In basic research, we have to create those interfaces ourselves, or we will be stuck to the ice age before we know it.
Are there other events planned or what are the next steps?
Besides the workshop, we are starting to hire – there is a Ph.D. position for a Joint doctorate at the University of Padova and at Université Clermont Auvergne, call open until May 12 at the University of Padova; the student will work on MODE research. We are also starting our activities in two important use cases, the optimization of muon tomography detectors and the study of hybrid calorimeters. We are writing a white paper on the use of differentiable programming for detector design. And we are participating in a proposal to join the ELLIS society within a larger community of HEP and astro-HEP scientists. Finally, we are participating in competitive funding, to provide ourselves with the needed fuel for a long journey.
How can interested scientists join and benefit from MODE?
To join mode you only need to declare your genuine interest in our research plan and to devote a fraction of your research time to some of our activities, or propose others within our interests. We hold online meetings every month or so, and everybody is welcome to attend.
Further information
- MODE Collaboration, https://mode-collaboration.github.io
- Toward Machine Learning Optimization of Experimental Design: http://inspirehep.net/record/1850892
- MODE workshop: https://mode-collaboration.github.io/workshop/index.html
Tommaso Dorigo (Ph.D. 1999) is a particle physicist and machine learning expert who works as a First Researcher for the INFN and teaches Particle Physics and Data Analysis courses at the University of Padova, Italy. He participates to the CMS experiment at the CERN LHC collider, where he is a member of the Statistics Committee, which he chaired in the years of the Higgs boson discovery. In 2020 Dorigo founded and since then coordinates the MODE collaboration. He is an author of over 1600 peer-reviewed scientific publications, and is an editor of the Elsevier “Reviews in Physics” and “Physics Open” journals; since 2006 he has also run a popular blog, visited over 14 million times (http://www.science20.com/quantum_diaries_survivor).